DanceTrack: A Unique Challenge in Multi-Object Human Tracking
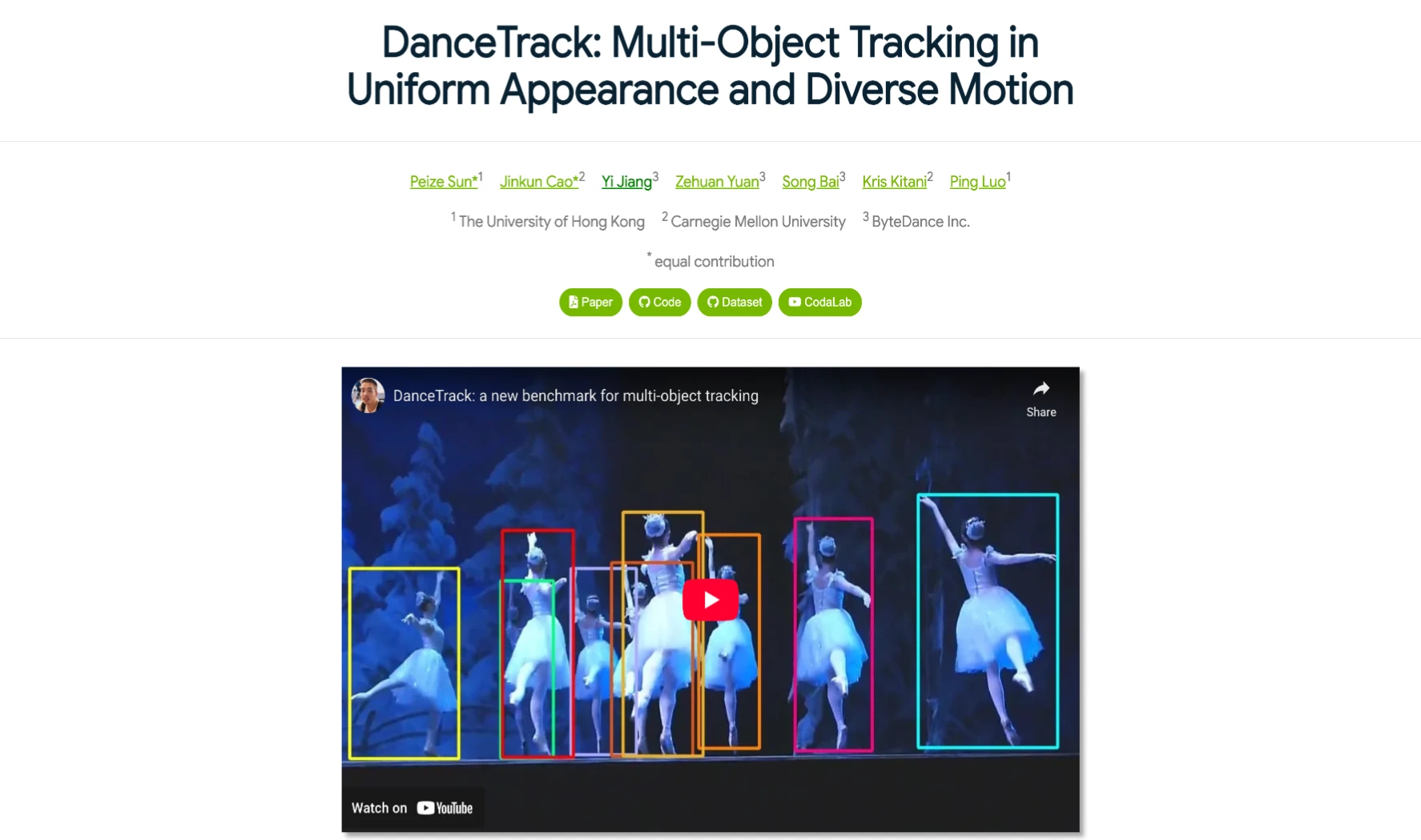
Tracking multiple humans in videos might sound simple at first—especially with today's deep learning tools—but when everyone looks the same and moves in unpredictable ways, it becomes a real challenge. That’s where DanceTrack comes in.
In this article, I’ll walk you through what DanceTrack is, why it matters, and how to use it effectively for multi-object tracking (MOT). I’ll also show you how to evaluate and visualize tracking results using the toolkit provided by the creators.
What is DanceTrack?
DanceTrack is a large-scale dataset designed specifically for tracking multiple humans in settings where appearance is almost identical and motion is highly dynamic. It addresses a key gap in existing datasets: most current benchmarks make tracking easier by having people wear different clothes or appear in distinct ways.
So, DanceTrack flips the usual assumptions by focusing on:
- Uniform Appearance: All people in a scene look almost the same.
- Diverse Motion: Subjects are constantly moving, changing positions, and showing extreme articulation, such as those seen in group dance or gymnastics.
This means we can't rely too much on visual appearance anymore. Instead, we have to focus more on motion, timing, and spatial analysis.
Dataset Overview
Property | Value |
---|---|
Total Videos | 100 |
Training Set | 40 videos |
Validation Set | 25 videos |
Test Set | 35 videos |
Unique Human Instances | 990 |
Average Video Length | 52.9 seconds |
Total Frames | 105,000 |
Annotated Bounding Boxes | 877,000 |
Frame Rate | 20 FPS |
Key Features of DanceTrack
Here are the elements that make DanceTrack stand out:
- Uniform Clothing: No more easy clues based on bright jackets or different hairstyles.
- Frequent Motion Shifts: People swap positions often, especially in choreographed dance scenes.
- Low-Light and Outdoor Scenes: Includes different lighting and background scenarios.
- Diverse Scene Types: From traditional group dancing to gymnastics with aggressive deformation.
Sample Scenes
DanceTrack includes a variety of environments such as:
- Outdoor dance sequences
- Dimly-lit rooms
- Large group performances
- Gymnastics routines with complex movement
These variations add to the difficulty of accurately tracking individuals.
Why DanceTrack is Different
Most tracking pipelines today use a two-step approach:
- Detection: Identify people in each frame.
- Re-identification (Re-ID): Match detected individuals across frames based on appearance.
This method works well on datasets where people wear different outfits or look noticeably different. But when appearance doesn't help, Re-ID breaks down.
DanceTrack brings a challenge where appearance similarity forces us to go beyond visual identity. It asks us to:
- Focus more on temporal consistency
- Use motion clues over appearance
- Create models that work in harder, more realistic scenarios
How to Use DanceTrack
DanceTrack is open-source and available for download. Here's how you can start using it.
Step 1: Get the Dataset
You can find all resources here:
Step 2: Structure Your Output
After running your tracker, you'll need to organize your results like this:
{DanceTrack ROOT} └── val └── TRACKER_NAME ├── dancetrack0001.txt ├── dancetrack0002.txt └── ...
Each .txt file should include one line per detection in the following format:
<frame>, <id>, <bb_left>, <bb_top>, <bb_width>, <bb_height>, <conf>, -1, -1, -1
These files are then used for evaluation.
Evaluation Guide
Let's walk through the evaluation process.
Step 1: Run Evaluation Script
Use the provided script:
python3 TrackEval/scripts/run_mot_challenge.py \ --SPLIT_TO_EVAL val \ --METRICS HOTA CLEAR Identity \ --GT_FOLDER dancetrack/val \ --SEQMAP_FILE dancetrack/val_seqmap.txt \ --SKIP_SPLIT_FOL True \ --TRACKERS_TO_EVAL '' \ --TRACKER_SUB_FOLDER '' \ --USE_PARALLEL True \ --NUM_PARALLEL_CORES 8 \ --PLOT_CURVES False \ --TRACKERS_FOLDER val/TRACKER_NAME
This will compute standard tracking metrics like:
- HOTA: Higher Order Tracking Accuracy
- MOTA: Multi-Object Tracking Accuracy
- IDF1: Identity F1 Score
- DetA: Detection Accuracy
- AssA: Association Accuracy
Sample Evaluation Result (Using ByteTrack)
Tracker | HOTA | DetA | AssA | MOTA | IDF1 |
---|---|---|---|---|---|
ByteTrack | 47.1 | 70.5 | 31.5 | 88.2 | 51.9 |
This shows that even popular models like ByteTrack take a hit in accuracy when tested on DanceTrack.
Visualizing Results
You can also create video visualizations of your tracking results.
Visualization Script
Run the following command:
python3 tools/txt2video_dance.py \ --img_path dancetrack \ --split val \ --tracker TRACKER_NAME
This script overlays your tracking boxes onto the video frames so you can visually inspect how well your tracker performs across scenes.
Using DanceTrack with ByteTrack
If you're using ByteTrack as your baseline, here's a quick checklist:
Download Trained Models
Available through two sources:
- Hugging Face (recommended)
- Baidu Drive (password: awew)
Training Instructions
Follow the setup guide on the official GitHub.
Evaluation Format
Ensure output .txt files are properly formatted.
Run Metrics
Use the provided Python script for evaluation.
Visual Analysis
Use the txt2video_dance.py script to create rendered video results.
Final Thoughts
DanceTrack isn't just another tracking dataset. It pushes us to think differently. In real-world applications like surveillance, sports analysis, or event monitoring, appearance can't always be trusted. People wear uniforms. Lighting changes. Motion becomes unpredictable.
With DanceTrack, we finally have a tool to test how well our tracking models work under these realistic challenges.
By removing the visual shortcuts and encouraging motion-based reasoning, it opens new areas of development in multi-human tracking. If you're working in computer vision or robotics, this dataset is worth a look.