Omnihuman1 Review: AI-Generated Human Video Framework
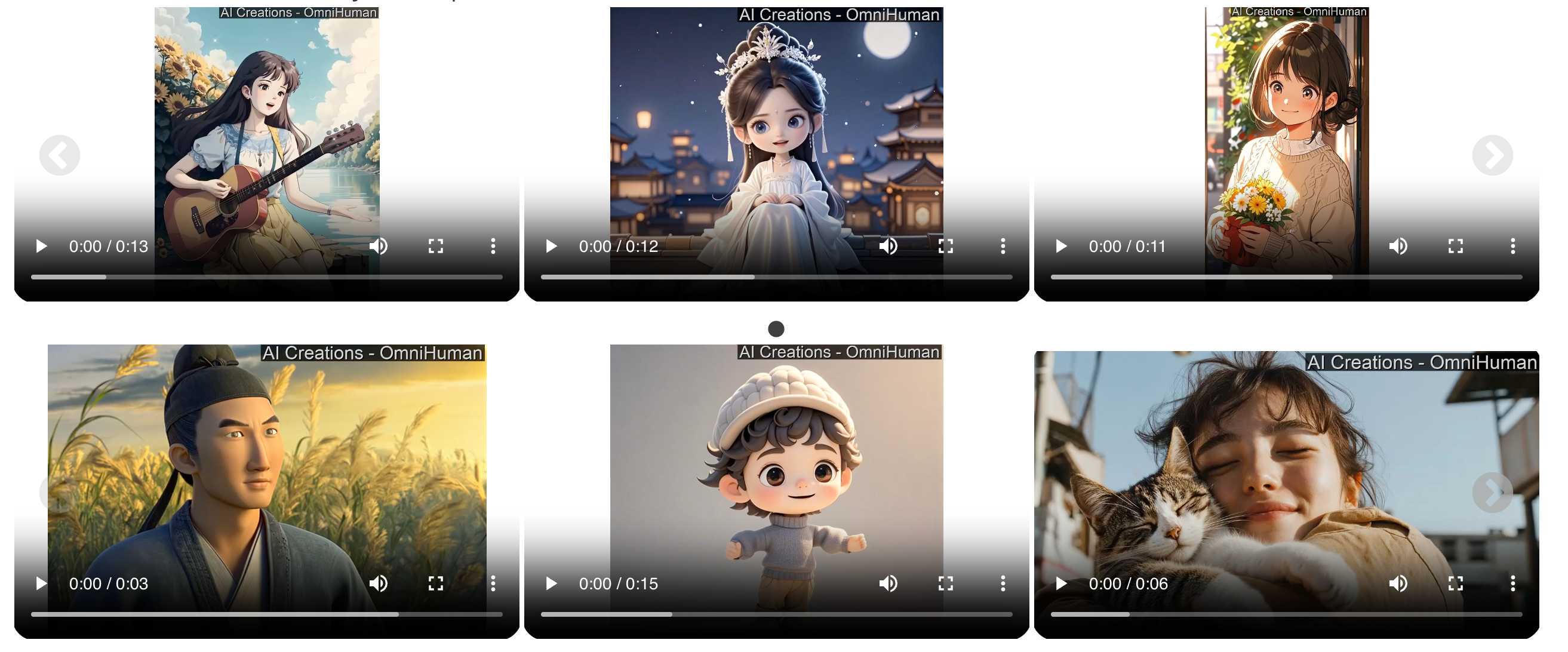
Introduction
In this article, I will provide a detailed overview of Omnihuman, an AI-driven human video generation framework. Omnihuman is an end-to-end, multimodal-conditioned video generation model capable of creating realistic human videos from a single image and motion signals like audio or video. I will also showcase some sample videos and explain the architecture of this incredible technology in simple terms.
Omnihuman in Action
Let’s look at some sample videos generated by Omnihuman.
The model can generate singing, talking, and various human movements seamlessly.
For instance, one video features a person singing with natural hair movement and minimal facial distortion—a significant improvement over other models in its category.
The eye movements, facial expressions, and fluidity of the generated characters make it clear that Omnihuman is taking video generation to another level.
The model can generate singing, talking, and various human movements seamlessly.
The model supports various aspect ratios and can work with both color and black-and-white images.
The diversity in outputs extends to anime, cartoons, and different human poses.
Due to YouTube restrictions, I’m not playing the music, but you can imagine how realistic these videos look. You can check the links in the video description to explore more.
Key Features of Omnihuman
1. Realistic Human Videos from Weak Inputs
Omnihuman can generate highly realistic human videos using minimal input signals like audio. This means that even with limited data, the model produces clear and lifelike results.
2. Supports Any Aspect Ratio
Unlike many other models, Omnihuman accepts input images of any aspect ratio, making it highly flexible for different video formats.
3. Accurate Facial and Body Movement
The model achieves high-quality motion synthesis, ensuring that generated videos exhibit natural expressions and body movements.
4. Multimodal Training Support
Omnihuman is trained using three different motion-related conditions:
- Text-based motion
- Audio-based motion
- Pose-based motion
By using a combination of these, the model enhances its ability to produce fluid and natural human animations.
Training Strategy: Omni-Condition Training
Omnihuman employs a unique training method known as Omni-Condition Training, which follows two main principles:
1. Weaker Conditions Strengthen Stronger Tasks
The model leverages data from weaker-conditioned tasks to scale up data during training. This improves learning efficiency and overall model accuracy.
2. Progressive Multi-Stage Training
The training process gradually introduces stronger conditions to refine the model’s capabilities. The stronger the condition, the lower the training ratio, optimizing performance without unnecessary resource consumption.
By following this approach, Omnihuman overcomes data scaling challenges, leading to significant improvements in motion learning and output diversity.
Model Architecture
Omnihuman's architecture consists of two core components:
1. Omnihuman Model
This model is based on a diffusion transformer architecture, supporting simultaneous conditioning with multiple modalities, including:
- Text
- Audio
- Pose data
2. Omni-Condition Training Strategy
This strategy progressively refines motion generation by leveraging mixed data sources. The mixed-condition training allows the model to improve continuously as it processes more data.
The facial and hair movement quality in the generated videos is incredibly detailed, with minimal blurring. While there is still room for improvement, Omnihuman already delivers impressive results.
Ethical Considerations
One notable aspect of Omnihuman is its commitment to addressing ethical concerns. The developers have implemented safeguards to prevent misuse, ensuring that AI-generated videos do not lead to unethical applications. This focus on responsible AI use is a welcome step toward maintaining integrity in video generation technologies.
Final Thoughts
Omnihuman is shaping up to be an impressive human video generation model. The realism in facial expressions, natural motion patterns, and multimodal support make it stand out from existing models.
That being said, AI-generated videos are still evolving, and there’s always room for further refinement. As researchers continue to enhance the model, we can expect even greater improvements in realism and flexibility.