MagicVideo-V2: Multi-Stage High-Aesthetic Video Generation
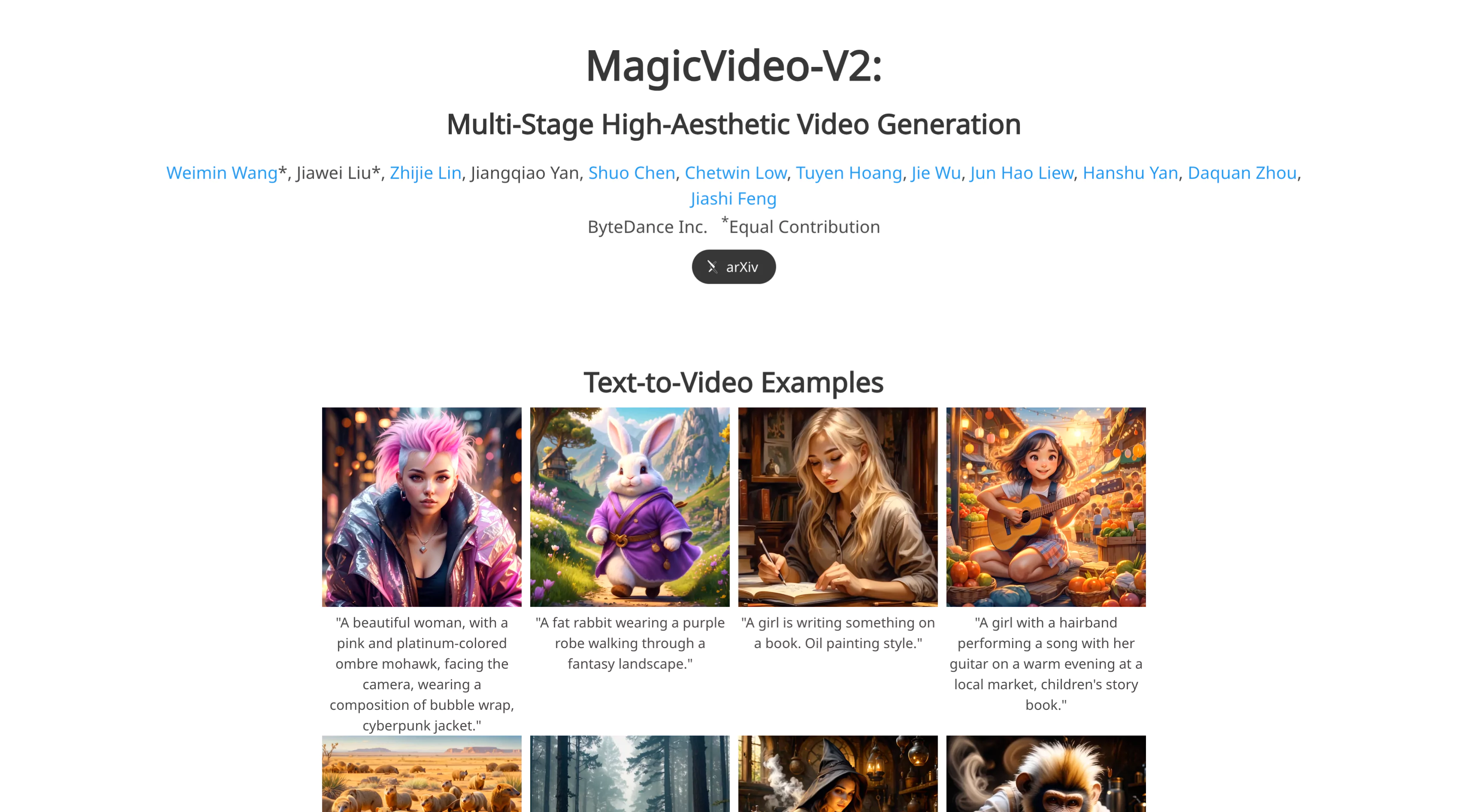
If you've ever tried turning a text prompt into a visually stunning video, you probably know the struggle. The output often lacks fluidity, consistency, or just doesn't look good enough.
That’s where MagicVideo-V2 comes in — a thoughtful and well-structured multi-stage system that helps generate visually appealing videos starting from a simple text description.
The rise of diffusion-based models has brought noticeable growth in the field of text-to-video (T2V) generation, and MagicVideo-V2 takes full advantage of this trend by introducing a combined framework.
It uses a sequence of components — from text-to-image to image-to-video, video enhancement, and finally, video interpolation — to make the whole process not just functional, but truly beautiful.
What is MagicVideo V2?
MagicVideo-V2 is a multi-step pipeline that generates high-quality, aesthetically pleasing videos from a text prompt. Unlike single-stage models, it breaks the process into smaller, more manageable phases, each refining the results from the previous stage.
This system uses the following modules in sequence:
- T2I (Text-to-Image): Creates an image based on the text prompt.
- I2V (Image-to-Video): Expands the image into low-resolution video keyframes.
- V2V (Video-to-Video): Enhances the resolution and visual details.
- VFI (Video Frame Interpolation): Smooths out the video motion by filling in the gaps between frames.
MagicVideo-V2 Overview
Module Name | Function | Input | Output |
---|---|---|---|
T2I | Text to image | Text prompt | Initial square image (1024x1024) |
I2V | Image to video keyframes | Image + Text | Low-res keyframe sequence |
V2V | Video enhancement | Keyframes | High-res video |
VFI | Frame interpolation | High-res video | Smooth and fluid motion |
Key Features of MagicVideo-V2
- Multi-module Architecture – Each step improves the results from the previous one.
- Internal Diffusion Model – The T2I module uses a custom diffusion model for high visual aesthetics.
- Motion Module – I2V incorporates a motion-aware system that was trained with human feedback.
- Cross Attention Conditioning – Enhances image fidelity and keeps frame details intact.
- ControlNet Integration – Provides better spatial conditioning using RGB values from reference images.
- Noise Prior Strategy – Ensures the generated frames maintain a coherent layout and structure.
- Joint Image-Video Training – Improves quality by treating single-frame images as videos.
- VFI Module with Deformable Convolution – Adds smoother transitions and more natural movement between frames.
How MagicVideo-V2 Works – Step-by-Step
Let’s go through each module one by one to understand the complete process.
1. Text-to-Image (T2I)
The process kicks off with the T2I module. Here's what it does:
- Takes a text prompt and creates a 1024x1024 image.
- This image represents both the theme and aesthetic of the final video.
- A custom in-house diffusion model is used to produce visually rich and artistically composed outputs.
2. Image-to-Video (I2V)
Once we have the image, we move to the I2V module.
- Built on a model known as High Aesthetic SD 1.5.
- Uses human feedback during training to enhance output.
- Includes a motion module and appearance encoder.
- Integrates the image prompt separately from the text prompt using cross-attention.
- Employs a latent noise prior to preserve image layout.
- Adds a ControlNet module to extract RGB values and apply them across video frames.
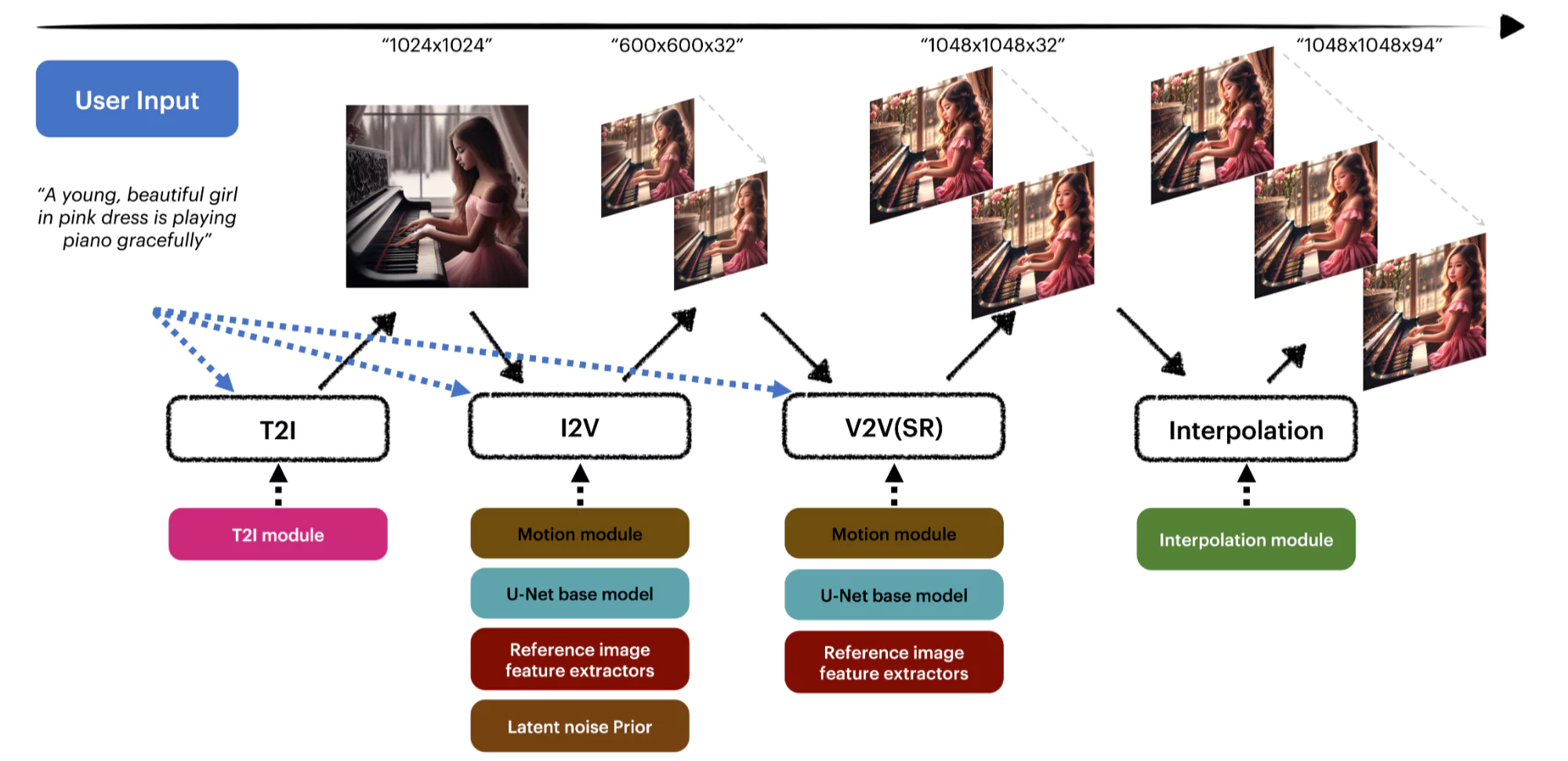
- Images treated as 1-frame videos for training.
- Allows the model to benefit from internal high-quality image datasets.
- This enhances frame quality and makes up for the smaller volume of video training data.
3. Video-to-Video (V2V)
The V2V module is where the low-res keyframes get a visual makeover.
- Shares the same base design and spatial layers as I2V.
- Uses a separately fine-tuned motion module specifically for video upscaling.
- Maintains layout by using the appearance encoder and ControlNet again.
- Helps guide high-resolution frame generation with fewer structural errors.
4. Video Frame Interpolation (VFI)
The final step is all about motion fluidity.
- Uses a GAN-based VFI module developed internally.
- Features an Enhanced Deformable Separable Convolution (EDSC) head.
- Paired with a VQ-GAN-like autoencoder.
- Includes a pre-trained lightweight interpolation model to add more frame consistency and natural motion.
How to Use MagicVideo-V2?
Although the paper doesn’t mention a public API or web interface, here’s how a typical usage flow would look for researchers or developers integrating this system:
Step-by-Step Guide
- Input a Text Prompt
Example: “A fox walking through a snowy forest at dusk.” - Generate the Initial Image
T2I model produces a 1024x1024 reference image matching the text. - Feed Image to I2V Module
Creates low-resolution video keyframes.
Motion is applied using internal models trained with aesthetic and layout constraints. - Refine with V2V Module
Upscales the keyframes to higher resolutions.
Fixes any artifacts or inconsistencies introduced in earlier steps. - Smooth Out with VFI
Generates in-between frames.
Ensures the final video has fluid, believable motion. - Export the Final Video
Output is a visually pleasing, high-resolution animation.
Human Evaluation and Experiment Results
To validate MagicVideo-V2, a series of human evaluation experiments were conducted.
Experiment Design
Participants: 61 human evaluators.
Comparison Count: 500 pairs of videos.
Task: Compare videos side-by-side — one from MagicVideo-V2 and the other from a competing model.
Text Prompt: Same for both videos in each pair.
Evaluation Criteria
- Frame quality and visual appeal
- Motion consistency
- Structural integrity (errors, glitches, etc.)
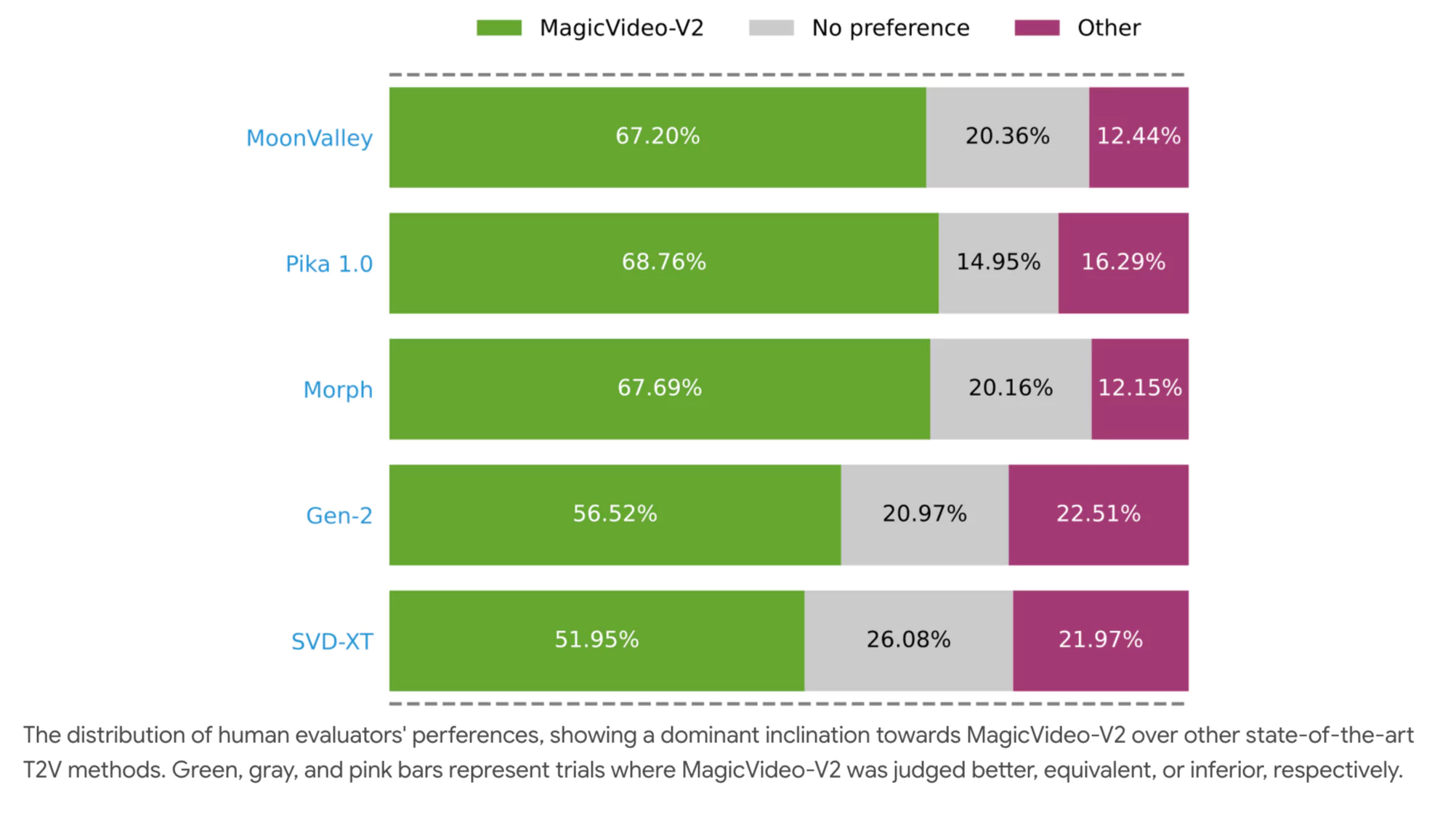
Rating Options
- Good – Preferred MagicVideo-V2
- Same – No preference
- Bad – Preferred competing video
Results Summary
Here’s a simplified table of what evaluators concluded:
Evaluation Result | % of Cases |
---|---|
MagicVideo-V2 Preferred | High majority |
No Preference | Moderate |
Competitor Preferred | Low |
The findings showed a clear edge for MagicVideo-V2 in visual quality and motion coherence.
In addition to the test results, they showcased qualitative samples, highlighting how the I2V and V2V modules corrected imperfections and brought more clarity to the final videos.
MagicVideo-V2 Example Videos
"A beautiful woman, with a pink and platinum-colored ombre mohawk, facing the camera, wearing a composition of bubble wrap, cyberpunk jacket."
"A fat rabbit wearing a purple robe walking through a fantasy landscape."
"A girl is writing something on a book. Oil painting style."
"A girl with a hairband performing a song with her guitar on a warm evening at a local market, children's story book."
Pros and Cons
Here’s a balanced look at the strengths and limitations:
- ✅ Pros
- Produces visually rich and stylistically aligned videos
- Modular approach allows for better refinement at each step
- Works well with limited video data thanks to image-video joint training
- ControlNet and noise prior techniques maintain visual consistency
- Final output has smoother and more natural motion
- ❌ Cons
- The process is relatively more complex than single-shot models
- Training and running the entire pipeline requires significant resources
- The system still depends on high-quality text prompts and reference images to achieve the best results
Summary and Final Thoughts
MagicVideo-V2 offers a well-structured pipeline for generating high-aesthetic videos from just a text prompt. Instead of relying on a single monolithic model, this method smartly splits the job into several focused modules. Each component does its part — from crafting the image to building motion, improving resolution, and smoothing out movement.
Thanks to techniques like ControlNet integration, noise prior adjustments, and joint training, the framework manages to produce outputs that not only look good but are also more coherent and fluid over time.
In my opinion, MagicVideo-V2 sets a high bar in text-to-video generation by showing that modular design, trained with real-world feedback, can deliver consistent and beautiful video outputs.
For more detailed information and updates, visit the official MagicVideo-V2 website by Click here.