DreamActor-M1 by ByteDance
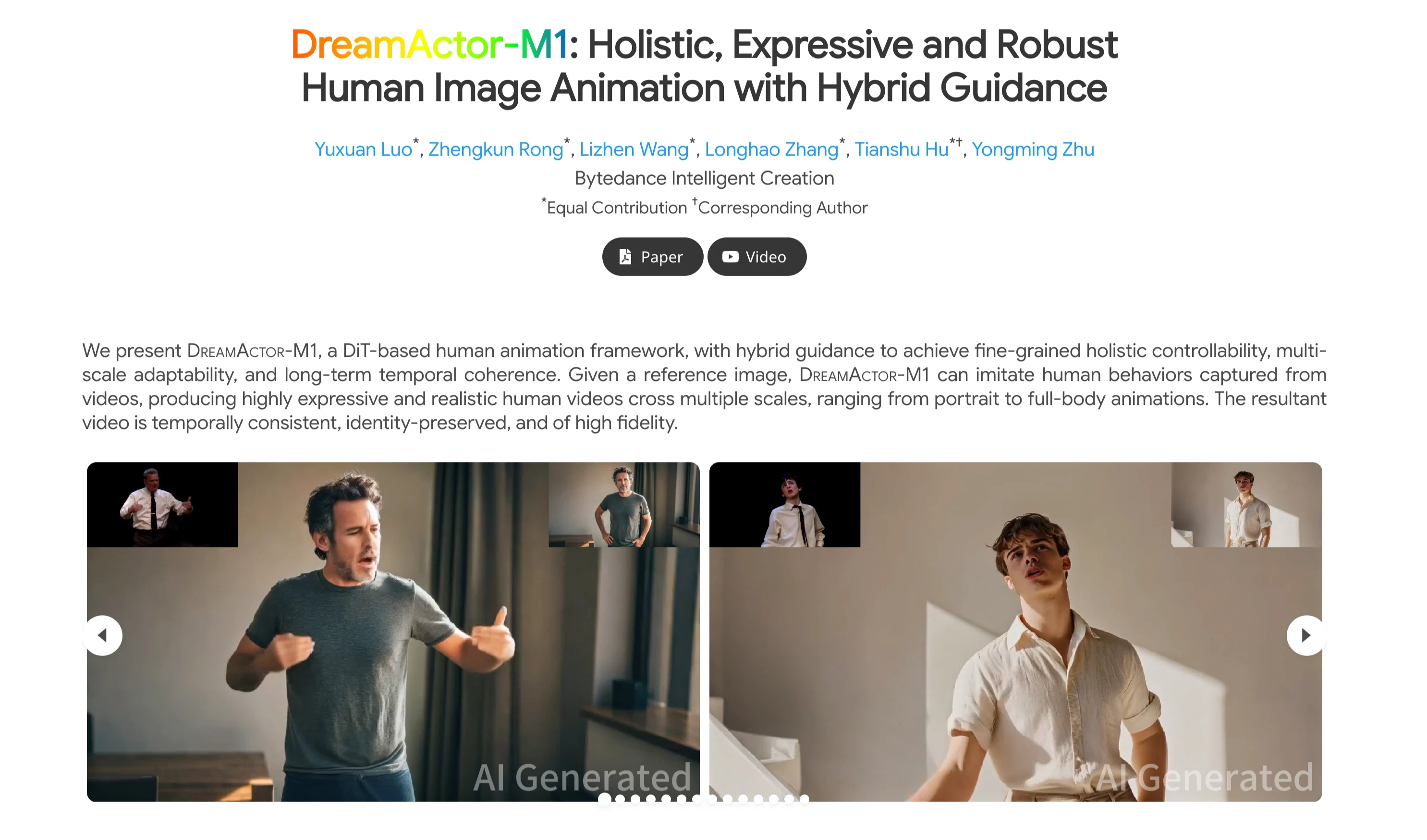
When I first saw the video demo of DreamActor-M1 by ByteDance, I was stunned. I had a simple reference image of a person and a driving video clip. And what DreamActor-M1 produced was something hard to ignore—it replaced the person in the video with the face and expressions from the image.
From facial expression to full body movement, everything looked so real, like it was acted out by the person in the reference photo.
What is DreamActor-M1 AI?
DreamActor-M1 is a video generation model developed by ByteDance. It creates videos that imitate human behavior by using just a single image as a reference. You provide the system with a photo and a video (called the "driving video"). The model then generates a new video where the subject in the image appears to be acting out the actions, expressions, and motions seen in the original video.
What really sets it apart is that it doesn’t just animate a face—it can animate a full body. It works well with simple portrait references and adapts them into full-body motions while keeping things smooth, clear, and consistent.
DreamActor-M1 Overview
Element | Description |
---|---|
Input | A single image and a video |
Output | A new video showing the image person doing the actions from the input video |
Identity Preservation | Maintains facial features and body structure |
Motion Handling | Head, face, and full-body gestures |
Temporal Consistency | Frames stay consistent in appearance and movement |
Model Type | Diffusion Image Transformer |
Application | Video creation, character animation, digital avatars |
Key Features
1. Image-to-Video Animation
Just one reference image is enough. The model converts that static image into a moving, expressive version. You can go from portrait-only to full-body with smooth results.
2. Motion Adaptation
It accurately follows the actions in the driving video. Includes face, head, torso, arms, and legs—all animated in sync. Facial expressions match the tone and speech of the video.
3. High Quality and Detail
Videos are generated in high detail. Maintains consistency in features throughout the clip. Suitable for portraits and full-body sequences.
4. Scalable Performance
Handles animations across different sizes: face-only, half-body, or full-body. Adapts smoothly regardless of the size or complexity of the motion.
5. Stable Output Across Frames
Temporal coherence is one of the biggest strengths. No flickering or unnatural transitions. Each frame flows into the next with visual accuracy.
Real Demos: What DreamActor-M1 Looks Like
The official demo showcases several scenarios that demonstrate the model's capabilities:
A reference image of a person speaking is used to animate an entire video of someone delivering a monologue. In another demo, the model starts with just a portrait and still delivers accurate body gestures and speech patterns.
It manages to preserve the identity of the original image across full-body actions.
"Why do I have to listen to you when you have zero to say because I'm young?"
The generated video perfectly aligns this audio with facial expressions and posture—even though it all began from a still image.
"I really want it and I want to do it well."
Again, full synchronization of movement and emotion is achieved using DreamActor-M1.
How to Use DreamActor-M1?
Although it's not publicly available yet, here's how it would generally work if you had access:
Step 1: Prepare Your Inputs
- Reference Image: One high-quality image of the person.
- Driving Video: A video of someone performing the motion or delivering a speech.
Step 2: Motion Extraction
The system extracts:
- Body skeleton
- Head movement
- Facial expressions
Step 3: Combine Inputs
Both the image and motion signals are passed to the trained DreamActor-M1 model.
Step 4: Generate Output
The system processes all inputs through its transformer-based architecture.
It produces a new video with your reference image mapped onto the actions.
DreamActor-M1 Architecture Overview
Here's a simplified breakdown of the architecture:
Component | Role |
---|---|
Pose Encoder | Converts body and head movement into pose latent |
3D VAE | Encodes short video clips into video latent |
Face Motion Encoder | Extracts facial expressions |
Reference Token Branch | Shares weights with noise token, adds identity detail |
Diffusion Transformer | Takes noise token and other inputs, generates final video |
Attention Layers | Ensure correct alignment of face, body, and visual appearance |
How Does DreamActor-M1 Work?
Let's break it into Training Phase and Inference Phase to make it easier to follow.
Training Phase
Here's what happens during the training stage:
- Pose Extraction
- Body skeletons and head shapes are pulled from the input driving video
- These are passed through a pose encoder to become "pose latent"
- Video Encoding
- A section of the driving video is encoded using a 3D Variational Autoencoder (VAE)
- This gives us the "video latent"
- Facial Expression Encoding
- Face motions are extracted using a separate face motion encoder
- The result is a set of facial motion tokens
- Reference Image Input
- A single frame or multiple frames are taken from the video to add appearance detail
- These form the "reference tokens"
- Integration with Diffusion Image Transformer (DIT)
- This model combines all tokens using cross attention and self attention techniques
- The face motion token is added through face attention
- Appearance information is merged with the noise token
- The output is then trained against the original video latent for accuracy
Inference Phase
Once training is done, the model is ready to generate videos. Here's what happens at inference:
- Reference Image Input
- You can give one or more images
- Multiple pseudo references are generated to give it more views of the same subject
- Driving Video Input
- The video provides the motion cues, such as body posture, head tilts, and lip sync
- Audio from the video also helps with timing and emotion
- Motion Signal Extraction
- Motion signals (facial and body) are pulled from the video
- These guide the generation process
- Final Synthesis
- The model combines appearance info from the image and motion cues from the video
- The output is a fully animated human video showing the subject in the reference image performing the actions in the video
Performance Benchmarks
ByteDance's comparison testing shows DreamActor-M1's superior performance across multiple metrics:
- Visual Accuracy: Demonstrates higher fidelity in full-body animation compared to existing tools
- Identity Preservation: Maintains more consistent facial features and personal characteristics than other portrait animation models
- Motion Quality: Produces smoother, more natural transitions between frames
- Temporal Coherence: Shows significant improvement in frame-to-frame consistency, reducing artifacts and glitches
These benchmarks establish DreamActor-M1 as a leading solution in the video animation space, particularly for full-body motion synthesis.
Final Thoughts
DreamActor-M1 is pushing the limits of what’s possible with video generation from images. From facial expressions to full body movement, everything is stitched together with such consistency that it's nearly impossible to tell it’s AI-generated.
Although it's not publicly available, the technology behind it is already setting new benchmarks. We need to be careful about how such tools are used, but there's no denying the quality it delivers.